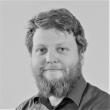
Research assistant, Loge
Tim Franken is a PhD student at the chair thermodynamics and thermal process engineering at Brandenburg University of Technology in Cottbus, Germany. He studied at the universities in Cologne and in Cottbus and finished with a master’s degree in mechanical engineering and energy technology in 2014. His research focus is zero-dimensional and three-dimensional combustion modeling of compression ignition and spark ignition engines. Currently, he is working on the project “water injection in turbocharged spark-ignited engines” funded by German association of combustion engines (FVV) with the aim to understand water effects on combustion and emission formation using 3D CFD simulation.
Speeches
Highly fuel-efficient Diesel engines, combined with effective exhaust aftertreatment systems, enable an economic and low-emission operation of heavy-duty vehicles. The challenge of its development arises from the present engine complexity, which is expected to increase even more in the future. The approved method of test bench measurements is stretched to its limits, because of the high demand for large parameter variations. The introduction of a physics-based quasi-dimensional stochastic reactor model combined with tabulated chemistry enables the simulation-supported development of these Diesel engines. The stochastic reactor model mimics mixture and temperature inhomogeneities induced by turbulence, direct injection and heat transfer. Thus, it is possible to improve the prediction of NOx emissions compared to common mean-value models. To reduce the number of designs to be evaluated during the simulation-based multi-objective optimization, genetic algorithms are proven to be an effective tool. Based on an initial set of designs, the algorithm aims to evolve the designs to find the best parameters for the given constraints and targets. The extension by metamodels improves the prediction of the best possible Pareto-front, while the time of optimization is kept low. This work presents a methodology to couple the stochastic reactor model and the multi-objective genetic algorithm. First, the stochastic reactor model is calibrated for 10 medium and high load operating points at low engine speeds. Second, each operating point is optimized to find the lowest fuel consumption and specific NOx emissions. Further, it was ensured that the maximum peak cylinder pressure and turbine inlet temperature are not exceeded. This enables a safe operation of the engine and exhaust aftertreatment system under the optimized conditions. The results reveal two major outcomes. First, the selection of the optimization and space filler algorithm is crucial to find the best possible Pareto-front. In this work, the NSGA-II genetic algorithm coupled with metamodels in conjunction with the Latin Hypercube space filler algorithm has proven to be the best choice. Second, the EGR rate and compression ratio are found to be the most effective measures to reduce fuel consumption and NOx emissions for the selected
engine design.